Introduction
In recent years, human genomic research has faced a significant challenge in characterizing the dark regions of the genome. These regions are difficult to assemble or align due to their low mappability, which results in few or no mappable reads and reads aligned with low mapping quality. Typically found in highly polymorphic or duplicated genomic areas, these dark regions remain elusive yet hold valuable insights crucial for advancing our understanding of the human genome.1,2
Another major challenge in human genomic research is reference bias, stemming from the limitations of using a single haploid human reference genome to represent the diversity of human sequences across populations.3 These two challenges are interconnected, as reference bias can exacerbate the difficulty of mapping reads in certain regions of the genome. The GRCh38 reference assembly4 attempted to address the reference bias problem, at least partially, by introducing alternative (alt) contigs included natively in the FASTA reference, especially in highly polymorphic regions, to better capture human genetic variation. These native alt contigs represent alternative paths to the corresponding regions in the primary contigs. However, handling these additional contigs requires special care, as mishandling them could introduce more errors than those the contigs are attempting to resolve. We explain this in a previous Illumina Genomics Research Hub article,5 where we show that DRAGEN has effectively utilized the relationship between these native alt contigs and the primary contigs in the GRCh38 reference to improve mapping accuracy. Initially, it used an alt-aware alignment liftover procedure, which was later replaced in version 3.9 by an alt-masking approach, further improving mapping accuracy.
However, these limited advancements haven't fully captured the majority of human variations. To tackle this, the community is now creating a collection of high-quality assemblies to serve as references. This effort is being led by several pangenome consortia, such as the Human Pangenome Reference Consortium (HPRC),6 the Chinese Pangenome Consortium (CPC),7 and the Arab Pangenome Reference (APR),8 aiming to create both global and ancestry-specific reference collections. In parallel with the efforts of the HPRC and other consortia, DRAGEN first introduced the concept of a multigenome mapper and pangenome reference in version 3.7, achieving drastic improvement in the mapping accuracy of Illumina reads in challenging genomic regions.9
Note: Historically we referred to the combination of both the method to perform mapping and the collection of samples used as reference as “multigenome (graph) reference.” Moving forward we will separate the method used to perform the mapping (multigenome mapper) from the collection of samples used as a reference for the mapping (pangenome reference) to better retrospectively describe the updates introduced on each version of DRAGEN.
Over time, as shown in Figure 1, DRAGEN has released several generations of multigenome mappers and pangenome references, each iteration further enhancing the accuracy of read mapping in difficult-to-map regions. Figure 2 illustrates the evolution of the reference used for mapping.
The first generation of the multigenome mapper, released in DRAGEN v3.7, expanded the native alt contigs with a set of population haplotypes extracted from a pangenome reference composed of 16 European samples. The introduction of the first-generation multigenome mapper led to a 47% reduction in SNP errors and a 24% reduction in indel errors compared to using the linear reference (including the native alt contigs).
In DRAGEN v4.2, we expanded our pangenome reference from 16 samples with European ancestry to 32 samples with ancestries from around the globe. This reduced ancestry bias, yielding further variant calling accuracy gains.
With the DRAGEN v4.3 release, we introduce the second-generation multigenome mapper, which enables the expansion of our pangenome reference from 32 to 128 population samples of 26 different ancestries around the globe (Figure 3). This pangenome reference is the most diverse set of population samples launched on DRAGEN yet!
In this article, we discuss the evolution of the multigenome mapping methodology using pangenome references and show the significant accuracy improvement both in the All Benchmark Regions and Difficult-to-Map Regions of the genome, with the second-generation multigenome mapper and the most recent 128-samples pangenome reference.
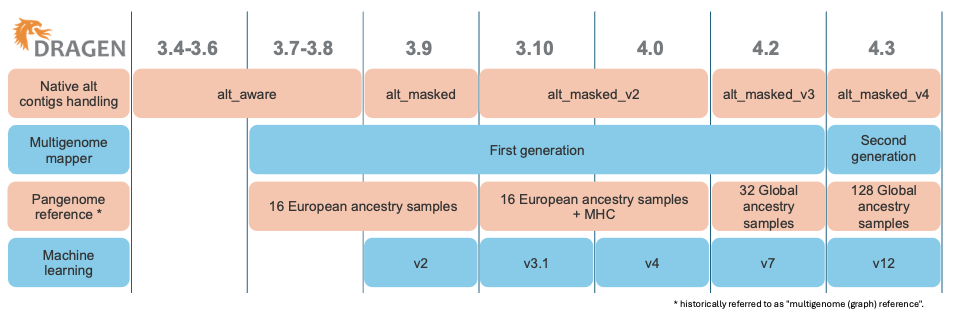
Figure 1: Evolution of the DRAGEN components including the native alt contigs handling, the multigenome mapper, the pangenome reference, and the machine learning.
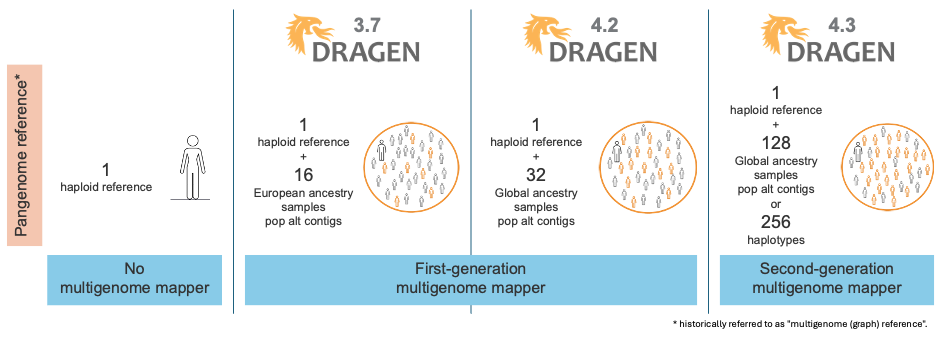
Figure 2: Evolution of the DRAGEN multigenome mapper—population aware and pangenome reference.
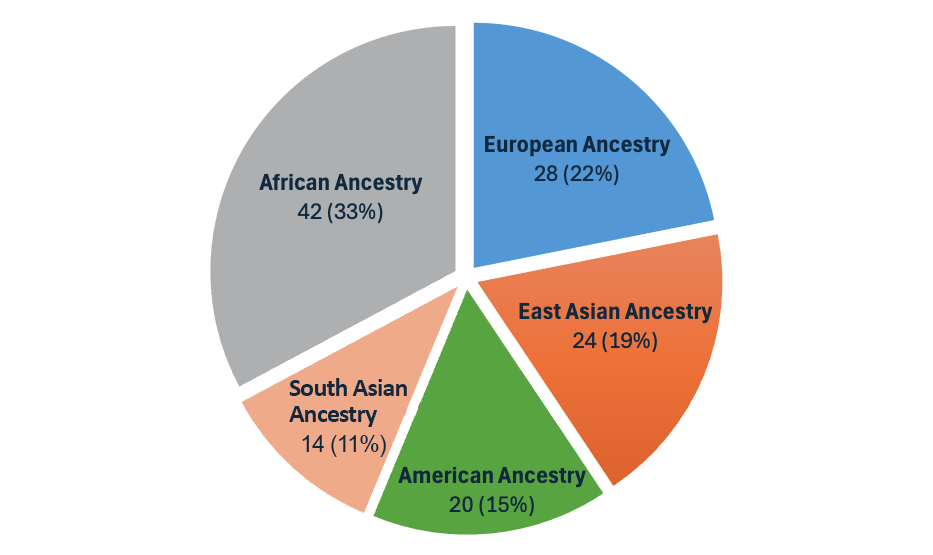
Figure 3: The pangenome reference in DRAGEN v4.3 is made up of 128 population samples from 26 ancestries around the world.
DRAGEN v4.3 brings the most accurate DRAGEN analysis ever
Figure 4 shows the accuracy of successive DRAGEN versions on the HG002 NIST sample for the v4.2.1 All Benchmark Regions,10 for SNPs and indels combined. DRAGEN accuracy has significantly improved over time, with an 83% error reduction over the past four years. The introduction of the first-generation multigenome mapper and 16-sample pangenome reference in version 3.7.5 led to the first steep error reduction. With the introduction of the second-generation multigenome mapper and 128-samples pangenome reference in DRAGEN v4.3, DRAGEN continues to set new standards for accuracy, and achieves another steep error reduction of 40% compared to DRAGEN v4.2.
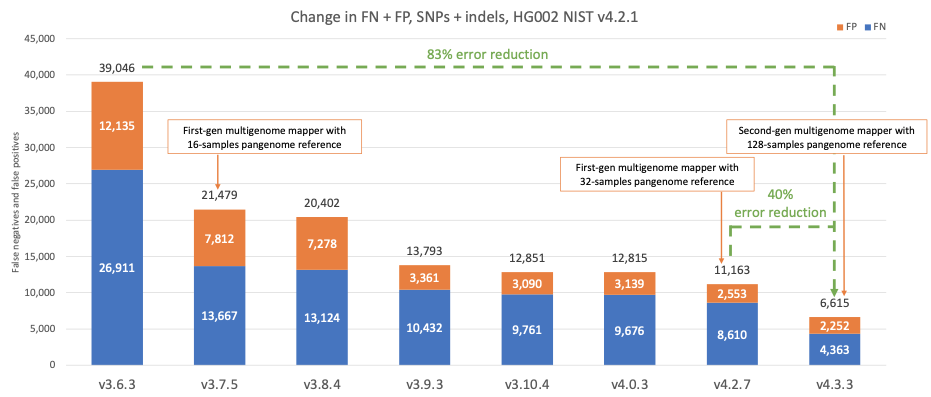
Figure 4: HG002 NIST v4.2.1 SNPs and indels false positives and false negatives error count on successive DRAGEN versions.
Benchmarking on the GIAB samples using the NIST v4.2.1 truth set
In Figure 5 we compare the accuracy of DRAGEN v4.3 to DRAGEN v4.2 and a third-party pipeline consisting of Giraffe11 1.54.0 for alignment and DeepVariant12 1.6.0 for small variant calling on the HG001–HG007 samples using Genome in a Bottle (GIAB)13 v4.2.1 on All Benchmark Regions.14 When compared to Giraffe-DeepVariant, DRAGEN v4.3 shows an average error reduction of 61.61% on combined SNPs and indels, with an average error reduction of 63.8% on SNPs and 53.53% on indels.
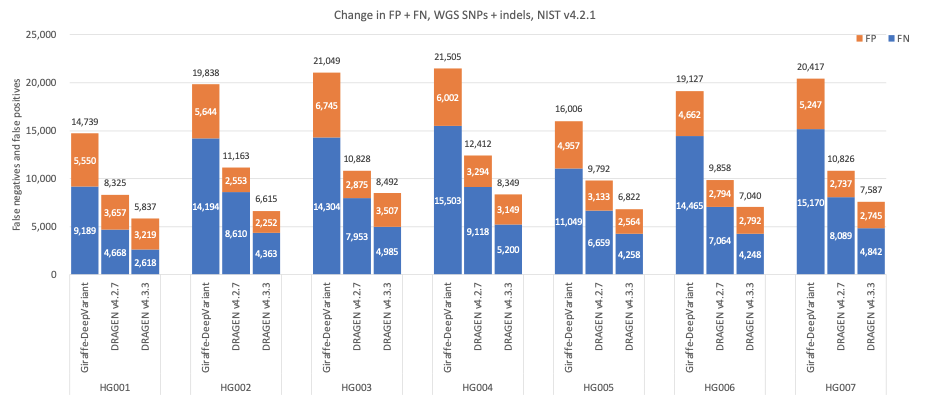
Figure 5: SNPs and indels cumulative error count on the seven GIAB samples (HG001–7), using the NIST v4.2.1 truth set, for DRAGEN v4.2, DRAGEN v4.3, and Giraffe-DeepVariant.
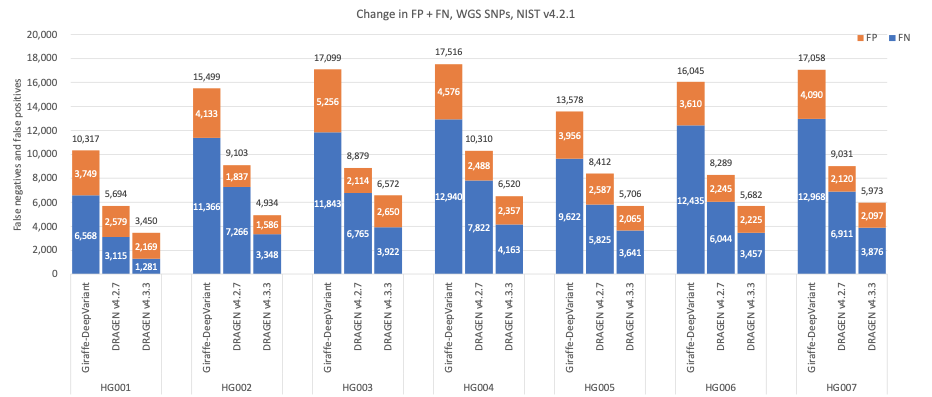
Figure 6: SNP cumulative error count on the seven GIAB samples (HG001–7), using the NIST v4.2.1 truth set, for DRAGEN v4.2, DRAGEN v4.3, and Giraffe-DeepVariant.
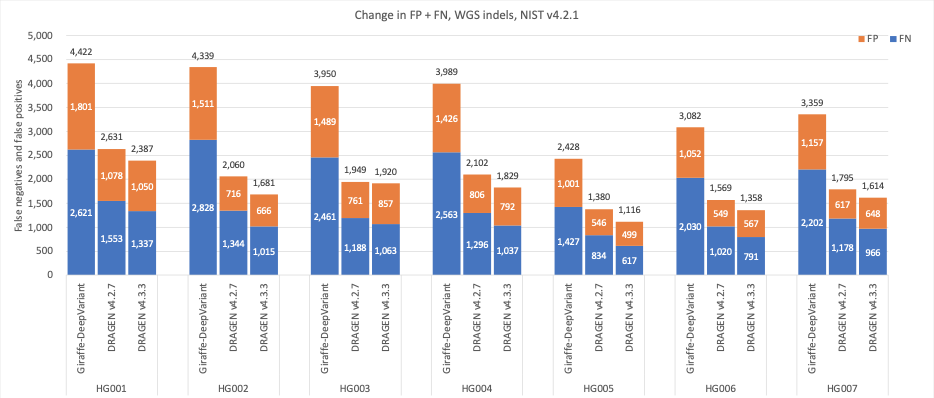
Figure 7: Indel cumulative error count on the seven GIAB samples (HG001–7), using the NIST v4.2.1 truth set, for DRAGEN v4.2, DRAGEN v4.3, and Giraffe-DeepVariant.
Benchmarking on the GIAB samples in the Difficult-to-Map Regions of the genome
Figure 8 shows the accuracy results in Difficult-to-Map Regions, as defined by the NIST,15,16 where DRAGEN 4.3 shows impressive gains on SNPs and indels accuracy with an average error reduction of 65.51% when compared to Giraffe-DeepVariant and a reduction of 37.77% when compared to DRAGEN 4.2.
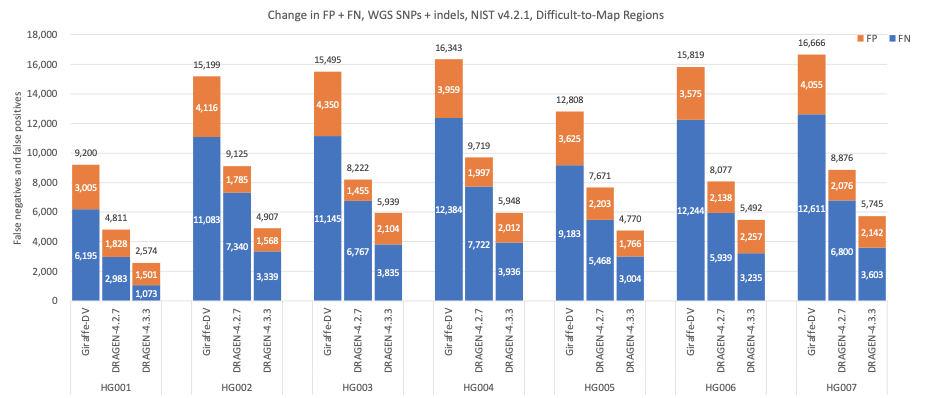
Figure 8: SNPs and indels cumulative error count on the seven GIAB samples (HG001–7), using the NIST v4.2.1 truth set intersected with the Difficult-to-Map Regions BED file, for DRAGEN v4.2, DRAGEN v4.3, and Giraffe-DeepVariant.
Benchmarking on the HG002 sample using the CMRG truth set
Figure 9 displays the accuracy of successive DRAGEN versions on the Challenging Medically Relevant Genes (CMRG) truth set17 for SNPs and indels combined. The total error count has continuously decreased over time, which is consistent with the error count evolution in NIST v4.2.1 All Benchmark Regions. The recent second-generation multigenome mapper with the 128-sample pangenome reference with DRAGEN v4.3 contributed an additional 15% reduction in total false positives and false negatives, resulting in 85 false negative calls being correctly detected across multiple genes. Both DRAGEN v4.2 and DRAGEN v4.3 outperform the Giraffe-DeepVariant pipeline. When compared to Giraffe-DeepVariant, DRAGEN v4.3 correctly detects more than 200 additional variants in medically relevant genes.
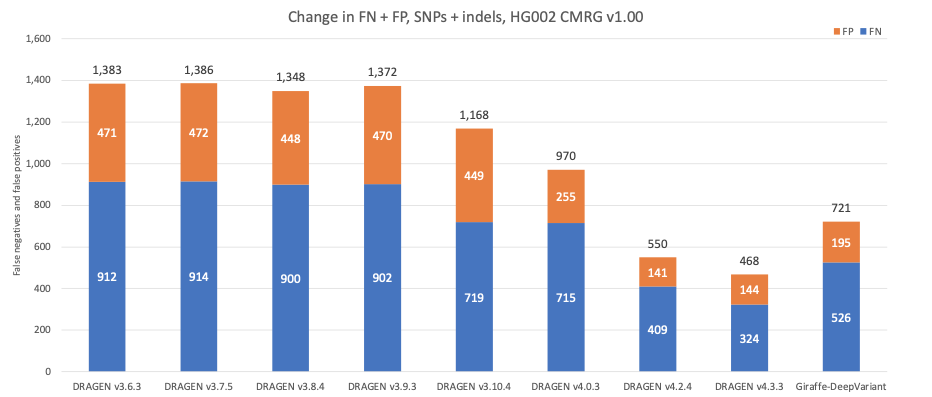
Figure 9: HG002 SNP and indel combined accuracy results in the CMRG truth set on successive DRAGEN versions and Giraffe-DeepVariant.
DRAGEN first-generation multigenome mapper methods
For the first generation of the DRAGEN multigenome mapper we derived population haplotypes in difficult-to-map regions of the genome from phased SNP and indel calls of the pangenome reference samples where low confidence or low population-allele frequency variants were excluded. In particular, we derived 32 population haplotypes from the 16 European ancestry samples and 64 population haplotypes from the 32 global ancestry samples.
DRAGEN second-generation multigenome mapper methods
The second generation of the multigenome mapper introduced in DRAGEN v4.3 builds upon the first-generation representation and addresses its main limitations. As the number of available population assemblies increases, the second-generation multigenome mapper was designed to improve scalability both vertically (number of samples from the population pangenome) and horizontally (whole-genome coverage). Additionally, it relies on a new structure that allows for long-range phasing, ensuring all population variants are phased.
This data structure uses a proprietary compression method to avoid storing redundant variant information shared among several haplotypes, recording only the set of locally distinct haplotypes while retaining all the long-range information. This approach enables efficient scaling when adding more haplotypes and improves scoring speed. It enabled a substantial increase in the haplotype panel size, from 64 in DRAGEN v4.2 to 256 in DRAGEN v4.3, including more ancestries.
Notably, compared to the first generation, which contained unphased SNPs as multi-base code, this new version phases all variants, enabling more accurate joint-scoring of paired-end mates across haplotypes.
The previous liftover system for alternate contigs is still used but is now focused on complex variants. The population SNPs are still encoded as multi-base code in the primary reference to improve the sensitivity of the seed-mapping process, but the alignment score is now computed using phased variant information from the new data structure.
Command line options
Command line options used for DRAGEN runs prior to version 4.3.3 are reported in a previous Illumina Genomics Research Hub article.19 The DRAGEN v4.3.3 runs used the following command line options, which are also explained in Table 1:
dragen \
--fastq-file1 <path-to-R1-fastq> \
--fastq-file2 <path-to-R2-fastq> \
--RGSM HG002 \
–RGID HG002 \
--ref-dir <path-to-reference-directory> \
--output-file-prefix HG002 \
--events-log-file dragen_events.csv \
--output-directory <path-to-output-directory> \
--generate-sa-tags true \
--enable-vcf-compression true \
--enable-variant-caller true \
--enable-map-align true \
--enable-map-align-output true \
--enable-sort true \
--enable-duplicate-marking true \
--enable-bam-indexing true
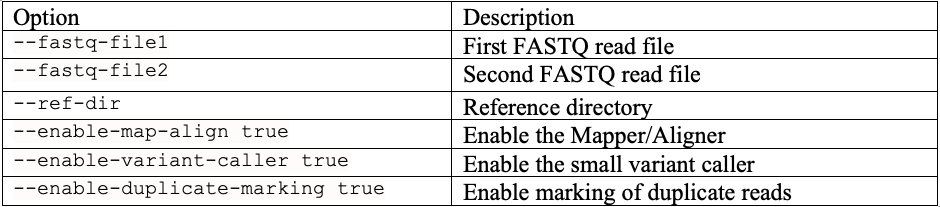
Table 1: DRAGEN command line options we used in our tests.
The DRAGEN v4.3 hg38-alt_masked.graph.cnv.hla.rna_v4 hash table is available from the DRAGEN Product Files page20 and the DRAGEN v4.3 ML model v12.0 is default and packaged with the DRAGEN executable.
Concordance with NIST truth is performed using the RTG toolkit as described in Nature Biotechnology.21 A comparison example command is as follows:
java \
-Djava.awt.headless=true \
-Dtalkback=false \
-Dusage=false \
-Xmx40g \
-jar RTG-3.9.1.jar vcfeval \
-b <truth>.vcf.gz \
-c <query>.vcf.gz \
-t <tmp_dir> \
-o <output_dir> \
--output-mode annotate \
--vcf-score-field QUAL \
--bed-regions <truth>.bed \
-Z \
--sample <truth sample>,<query sample> \
--ref-overlap
For all DRAGEN runs, we use the recommended <sample>.hard-filtered.vcf.gz VCF output file, which results in best f1-score measure.
When evaluating the Difficult-to-Map Regions we additionally included the --evaluation-regions <stratification>.bed flag to provide the stratification bed to the RTG toolkit.
For the Giraffe-based pipeline, we followed the recipe as specified in a DeepVariant-VG case study,22 using the hprc-v1.1-mc-grch38.d9 reference released on AWS.23 We aligned the reads using Giraffe v1.52.0. The command lines and parameters are as follows:
vg giraffe \
--progress \
--read-group "ID:1 LB:lib1 SM:HG002 PL:illumina PU:unit1" \
--sample "HG002" \
--prune-low-cplx \
--max-fragment-length 3000 \
--output-format bam \
-f <path-to-R1-fastq> \
-f <path-to-R2-fastq> \
-x hprc-v1.1-mc-grch38.d9.xg \
-Z hprc-v1.1-mc-grch38.d9.gbz \
-d hprc-v1.1-mc-grch38.d9.dist \
-m hprc-v1.1-mc-grch38.d9.min \
--ref-paths hprc-v1.1-mc-grch38.d9.ref_paths \
-t 32 > HG002.giraffe.grch38.d9.bam
Sort the output BAM with sambamba v0.8.1 and index with samtools v1.15.1:
sambamba sort \
-t 32 \
-o HG002.giraffe.grch38.d9.sort.bam \
HG002.giraffe.grch38.d9.bam
samtools index \
-@ 32 \
HG002.giraffe.grch38.d9.sort.bam
Variant calling using DeepVariant v1.6.0 with the following singularity command:
singularity run \
--bind "${INPUT_DIR}:/mnt/input,${REF_DIR}:/mnt/reference,${OUTPUT_DIR}:/mnt/output,${BIND_TMPDIR}:/tmp" \
deepvariant_1.6.0.sif \
/opt/deepvariant/bin/run_deepvariant \
--ref="/mnt/reference/hprc-v1.1-mc-grch38.d9.fa" \
--reads="/mnt/input/HG002.giraffe.grch38.d9.sort.bam" \
--model_type="WGS" \
--sample_name="HG002" \
--output_vcf="/mnt/output/HG002.vcf.gz" \
--output_gvcf="/mnt/output/HG002.g.vcf.gz" \
--make_examples_extra_args="min_mapping_quality=1,keep_legacy_allele_counter_behavior=true,normalize_reads=true" \
--haploid_contigs="chrX,chrY" \
--par_regions_bed="/mnt/reference/hprc-v1.1-mc-grch38.d9.par_regions.bed" \
--num_shards="40"
Summary
Through its various releases, DRAGEN has continually set new standards for accuracy by meticulously designing its components. Although this article highlights the new generation of DRAGEN multigenome mapping (second generation) based on the pangenome reference, other components, like machine learning and variant calling, have also been enhanced in DRAGEN v4.3, contributing to its high performance.
Learn more about the advancements in DRAGEN v4.3 on the Illumina Software Resources blog.
Overall, DRAGEN v4.3 demonstrates impressive accuracy in calling germline small variants also in the dark regions of the genome, consistently outperforming previous versions and third-party pipelines in tests on the NIST v4.2.1 All Benchmark Regions and Difficult-to-Map Regions, and the CMRG regions. Renowned for its accuracy, speed, scalability, ubiquity, and comprehensiveness, DRAGEN remains an essential tool for genomic analyses, providing researchers and scientists with a powerful resource for comprehensive and efficient genomic data analysis.
References
- Ebbert MTW, Jensen TD, Jansen-West K, et al. Systematic analysis of dark and camouflaged genes reveals disease-relevant genes hiding in plain sight. Genome Biol. 2019;20(1):97. doi:10.1186/s13059-019-1707-2
- Ryan NM, Corvin A. Investigating the dark-side of the genome: a barrier to human disease variant discovery? Biol Res. 2023;56(1):42. doi:10.1186/s40659-023-00455-0
- Miga KH, Wang T. The Need for a Human Pangenome Reference Sequence. Annu Rev Genomics Hum Genet. 2021;22:81-102. doi:10.1146/annurev-genom-120120-081921
- GRCh38 Full Analysis Set Plus Decoys HLA. ftp.1000genomes.ebi.ac.uk/vol1/ftp/technical/reference/GRCh38_reference_genome/GRCh38_full_analysis_set_plus_decoy_hla.fa
- Catreux S, Farrell F, Mehio R, et al. Demystifying the versions of GRCh38/hg38 reference genomes, how they are used in DRAGEN and their impact on accuracy. Illumina website. illumina.com/science/genomics-research/articles/dragen-demystifying-reference-genomes.html. Published December 9, 2021. Accessed July 18, 2024.
- Liao WW, Asri M, Ebler J, et al. A draft human pangenome reference. Nature. 2023;617(7960)312-324. doi:10.1038/s41586-023-05896-x
- Gao Y, Yang X, Chen H, et al. A pangenome reference of 36 Chinese populations. Nature. 2023;619(7968):112-121. doi:10.1038/s41586-023-06173-7
- Uddin M, Nassir N, Almarri M, et al. A draft Arab pangenome reference. Preprint. Research Square. October 2023. doi:10.21203/rs.3.rs-3490341/v1
- Catreux S, Jain V, Murray L, et al. DRAGEN sets new standard for data accuracy in PrecisionFDA benchmark data. Optimizing variant calling performance with Illumina machine learning and DRAGEN graph. Illumina website. illumina.com/science/genomics-research/articles/dragen-shines-again-precisionfda-truth-challenge-v2.html. Published January 12, 2022. Accessed July 18, 2024.
- GIAB HG002 v4.2.1 truth. ftp-trace.ncbi.nlm.nih.gov/ReferenceSamples/giab/release/AshkenazimTrio/HG002_NA24385_son/NISTv4.2.1/.
- Sirén J, Monlong J, Chang X, et al. Pangenomics enables genotyping of known structural variants in 5202 diverse genomes. Science. 2021;374(6574). doi:10.1126/science.abg8871
- Poplin R, Chang PC, Alexander D, et al. A universal SNP and small-indel variant caller using deep neural networks. Nat Biotechnol. 2018;36(10):983-987. doi:10.1038/nbt.4235
- Wagner J, Olson ND, Harris L, et al. Benchmarking challenging small variants with linked and long reads. Cell Genom. 2022;2(5):100128. doi:10.1016/j.xgen.2022.100128
- GIAB samples release. ftp-trace.ncbi.nlm.nih.gov/ReferenceSamples/giab/release/.
- Difficult-to-Map Regions BED file. ftp-trace.ncbi.nlm.nih.gov/ReferenceSamples/giab/release/genome-stratifications/v3.3/GRCh38@all/Union/GRCh38_alllowmapandsegdupregions.bed.gz.
- Olson ND, Wagner J, McDaniel J, et al. PrecisionFDA Truth Challenge V2: Calling variants from short and long reads in difficult-to-map regions. Cell Genom. 2022;2(5):100129. doi:10.1016/j.xgen.2022.100129
- Wagner J, Olson ND, Harris L, et al. Curated variation benchmarks for challenging medically relevant autosomal genes. Nat Biotechnol. 2022;40(5):672-680. doi:10.1038/s41587-021-01158-1
- Behera S, Catreux S, Rossi M, et al. Comprehensive and accurate genome analysis at scale using DRAGEN accelerated algorithms. Nat Biotechnol. 2024. Published 2024 Oct 25. doi:10.1038/s41587-024-02382-1
- Rossi M, Catreux S, Roddey C, et al. Unlocking the full potential of Illumina genomes: The journey to enhanced variant calling quality with DRAGEN informatics and high-quality sequencing. Illumina website. illumina.com/science/genomics-research/articles/CMRG_hg38.html. Published June 29, 2023. Accessed July 18, 2024.
- DRAGEN Bio-IT Platform Product Files. emea.support.illumina.com/sequencing/sequencing_software/dragen-bio-it-platform/product_files.html.
- Krusche P, Trigg L, Boutros PC, et al. Best practices for benchmarking germline small-variant calls in human genomes. Nat Biotechnol. 2019;37(5):555-560. doi:10.1038/s41587-019-0054-x
- Using graph genomes: VG Giraffe + DeepVariant case study. github.com/google/deepvariant/blob/0bba6a71b8b0c2046a3b01c0bda1a5a0d2b80fca/docs/deepvariant-vg-case-study.md.
- hprc-v1.1-mc-grch38. s3-us-west-2.amazonaws.com/human-pangenomics/index.html?prefix=pangenomes/freeze/freeze1/minigraph-cactus/hprc-v1.1-mc-grch38/.